Unlocking the Power of Data Labelling Service
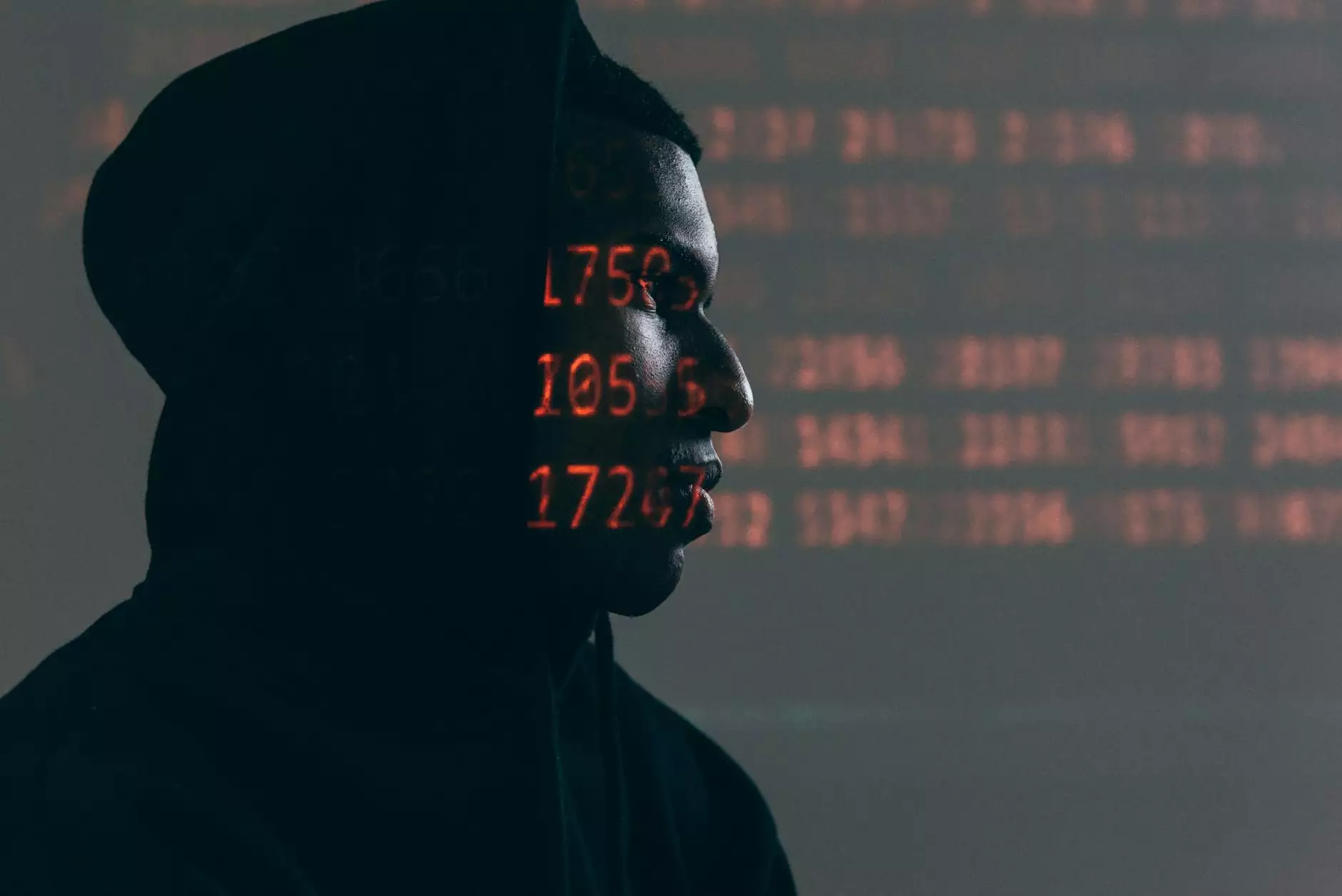
The world of machine learning and artificial intelligence (AI) is evolving at a breakneck speed. With an abundance of data generated each second, businesses face the pivotal challenge of harnessing this data effectively. At the core of this transformation lies a critical service: the data labelling service. This article delves deep into the significance, processes, and benefits of data labelling, illustrating how it can empower organizations to thrive in a data-driven economy.
Understanding Data Labelling
Data labelling is the process of classifying and annotating data to make it understandable by algorithms, particularly for training machine learning models. It involves marking images, texts, or audio files with relevant tags that denote their content. For instance, in image recognition, a photo of a cat may be labelled with "cat," representing the object within that image.
Types of Data Labelling
- Image Labelling: Identifying objects within images and bounding them with boxes.
- Text Labelling: Annotating textual data, such as identifying sentiments or classifying content.
- Audio Labelling: Tagging audio segments for speech recognition tasks.
- Video Labelling: Annotating sequences in video files to delineate objects or actions over time.
The Need for a Quality Data Labelling Service
In the realm of artificial intelligence, the saying "garbage in, garbage out" reigns true. The effectiveness of AI models heavily relies on the quality of the data fed into them. Here’s why a specialized data labelling service is crucial:
- Accuracy: It provides precise labels that are vital for training models that perform well.
- Scalability: Businesses can manage large datasets efficiently, ensuring consistent labelling across thousands of files.
- Domain Expertise: Access to trained professionals skilled in data annotation who understand the nuances of specific fields.
- Cost-Efficiency: Outsourcing data labelling reduces the costs associated with hiring full-time employees.
The Data Labelling Process
Implementing a data labelling service involves several systematic steps to ensure that the output is of the highest quality:
1. Data Collection
Initially, data must be gathered from various sources, including databases and live feeds. This data can be images, videos, text files, or audio recordings. Businesses need to determine the scope of the data required for their projects.
2. Choosing a Labelling Method
Depending on the project, businesses can opt for different labelling methodologies, such as:
- Manual Labelling: Human annotators carry out the labelling process, providing high accuracy but requiring more time.
- Automated Labelling: Using machine learning algorithms to make predictions that are then verified by humans for accuracy.
3. Annotation Guidelines
It is essential to develop clear annotation guidelines that detail how data should be labelled. This includes examples, common pitfalls, and specific instructions tailored to different types of data to ensure uniformity.
4. Quality Assurance
The integrity of labelled data is paramount. Implementing a quality assurance (QA) process where subsets of data are reviewed for accuracy ensures that the labels meet the required standards. This step can involve:
- Random Sampling: Regular checks on labeled data to ensure adherence to guidelines.
- Feedback Loops: Continuous improvement based on reviewer feedback and error analysis.
Benefits of Utilizing Professional Data Labelling Services
Professional data labelling services like KeyLabs.ai provide numerous benefits that can catalyze your business's success:
1. Enhanced Model Performance
With precise and accurate data annotations, machine learning models can achieve higher predictive accuracy and better performance metrics, leading to improved decision-making.
2. Time Efficiency
Outsourcing data labelling means that businesses can focus on core activities while experts handle the time-consuming process of annotation. This increased efficiency can lead to faster project turnaround times.
3. Access to Technology
Data labelling platforms often come equipped with advanced tools and technologies that modernize the labelling process, offering features like collaborative workspaces, workflow management, and real-time data handling.
4. Cost-Effective Solutions
By leveraging external data labelling service providers, businesses can significantly reduce operational costs associated with maintaining an internal labelling team or infrastructure.
Case Studies: Success Stories of Data Labelling Services
Numerous companies have reaped significant rewards from utilizing data labelling services. Here are a few notable case studies:
Case Study 1: E-commerce Product Recommendations
A leading e-commerce platform partnered with a data labelling service to tag thousands of product images and descriptions. By utilizing high-quality labels, they improved their recommendation engine's accuracy, resulting in a 30% increase in sales conversions.
Case Study 2: Autonomous Driving
A major automotive manufacturer used a data labelling service to annotate video footage from their autonomous vehicle prototypes, identifying various objects on the road. This service resulted in reduced accidents in their AI systems during testing phases and accelerated their product launch by months.
Best Practices for Choosing a Data Labelling Service
Selecting the right data labelling service is critical for ensuring the success of machine learning projects. Here are some best practices:
1. Assess Quality Assurance Processes
Ensure the provider has robust QA measures in place, including regular audits and feedback mechanisms.
2. Evaluate Scalability
Choose a service that can easily scale with your project as your data volume and complexity grows.
3. Look for Domain Expertise
Select a provider with experience in your industry to maximize the relevance and accuracy of the annotations.
4. Review Technology and Tools
Make sure the service uses cutting-edge technology and a seamless platform for collaboration, tracking, and management.
Conclusion: The Path Forward with Data Labelling
As businesses navigate the complexities of a data-driven landscape, the role of a data labelling service becomes more pronounced, becoming an essential element in the AI and machine learning ecosystems. By understanding the processes, benefits, and best practices of data labelling, organizations can position themselves to extract maximum value from their data investments.
KeyLabs.ai stands at the forefront of this transformation, offering top-tier data annotation tools and platforms tailored to meet the diverse needs of businesses today. By prioritizing quality and consistency in data labelling, we help our clients accelerate their AI initiatives and drive impactful results. Explore our services to unlock the true potential of your data and take your business to the next level.